How to Meet the Growing Energy Needs of AI
Generative AI uses a lot of energy. Can we balance AI's potential with the need for more power?
ChatGPT, Gemini and other generative AI tools have become mainstays in many of our day-to-day routines. In a recent survey, 65% of respondents reported that their organizations routinely use GenAI – nearly double the percentage than ten months earlier. It's already proving a force for good, allowing organizations to create personalized learning experiences, accelerate medical breakthroughs, optimizing energy grids, and more.
As GenAI cements its place in our lives, conversations are shifting from its utility to how we can meet the significant energy demand associated with it. Meeting that challenge calls for a greater mix of energy sources, from the development of nuclear energy to clean energy technologies like CCUS which can decarbonize existing power sources.
Unprecedented power, unprecedented energy demand
Popular GenAI tools are based on large language models (LLMs). More gigantic than large, these LLMs use neural networks with billions of parameters, which require vast amounts of computing power across multiple machines to train and use. Added to which, they are running 24 hours a day. All that energy use adds up quickly.
For example, it would take an average American household over 120 years to match the amount of energy used to train GPT-3. But it's not just training we need to consider. LLMs are also intensive to run: the energy required for one ChatGPT search is approximately ten times more than a Google search.
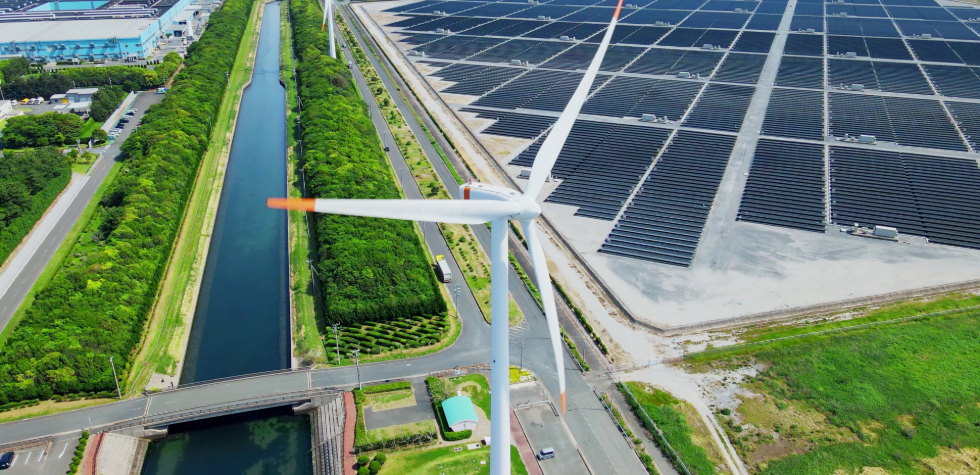
After years of a stable level of consumption, experts estimate that demand for power from data centers will grow by 160% by 2030. They suggest that AI may be responsible for an extraordinary 19% of data center power demand by 2028.
Those data centers are also resource-hungry, demanding large quantities of water. In 2021, before GenAI emerged into the public sphere, Google’s global data center fleet consumed approximately 4.3 billion gallons of water. The average Google data center used 450,000 gallons of water per day.
Mitigating strategies
To help AI realize its full potential, it is essential to develop strategies that address its energy demands while mitigating environmental impacts.
There is already some progress on this front. Many tech firms have turned to nuclear energy as a low-carbon power source for their AI operations. Microsoft signed an agreement in September to restart the mothballed Three Mile Island US nuclear power station to power AI data centers. Other Big Tech companies including Google and Amazon also plan to use nuclear power to power their AI data centers.
Improving more energy-efficient data centers is also essential. Innovations in cooling systems, including liquid cooling and advanced airflow management, can significantly reduce energy wastage. Microsoft has been exploring liquid cooling systems, which directly absorb and dissipate heat more efficiently than traditional air cooling, for its Azure data centers to handle the heat generated at its data centers. These innovations demonstrate that companies recognize the need to invest not only in AI, but in more efficient infrastructure and low-carbon energy sources to power it.
More broadly, the development of GenAI has also been cloaked in mystery, with little insight into the true energy cost of the latest LLMs. Encouraging transparency on energy use and emissions will help hold companies accountable, and balance technological progress with environmental preservation.
Striking this balance will require political will and collaboration across the public and private sectors. With regulation, investment, oversight and transparency, AI can serve as a catalyst for the transition to an energy system which meets our growing needs.